Personalization at scale requires companies to take on the challenges of technology and business in tandem, starting in the C-suite.
The true prize of modern marketing is delivering experiences that are both world class to the consumer and deliver value to the business. Personalization is a crucial weapon in the marketer’s arsenal to achieve that goal. That’s how businesses can deliver tailored recommendations, content, offers, and experiences, across all channels and devices, along the entire customer journey. Personalization at scale has the potential to create $1.7 trillion to $3 trillion in new value (Exhibit 1). Capturing this value will require mastering the technologies and addressing the organizational disconnects—all while forging trust with customers and protecting their data. But implementing and integrating the right technologies create significant complexity and call for a lot of coordination. The companies that overcome the barriers to personalization at scale are those that tackle both technology and business challenges in tandem, starting with the CMO and CTO/CIO working together closely.
The 4Ds that drive personalization at scale
Personalization at scale relies on an organization’s ability to orchestrate the 4Ds—Data, Decisioning, Design, and Distribution. Addressing the technology in each of these Ds is the key to unlocking the full power of personalization.
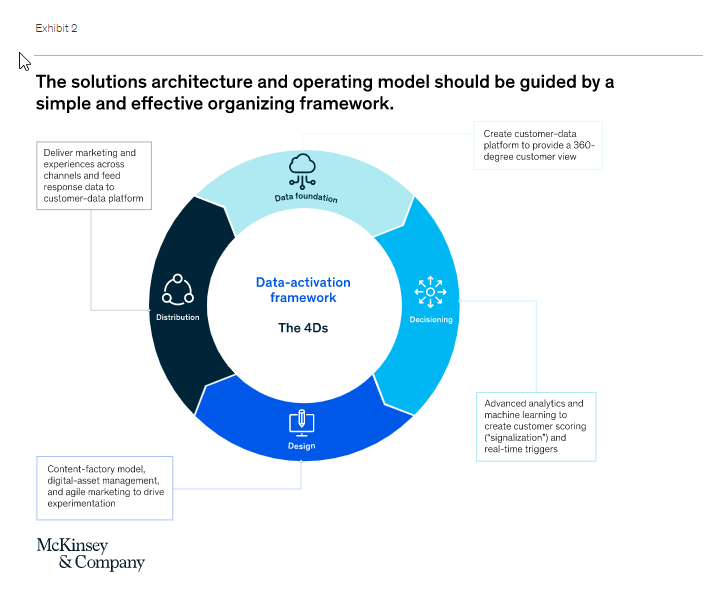
Data
Challenge: The data required for effective personalization is trapped in silos.
Solution: Integrate a customer-data platform and data-management platform, augmented with identity-resolution platforms to unify data and make it available across channels for activation.
Data must be centralized and made available so activity in one channel can immediately support engagement in another in real time or near–real time. For this to happen, three critical data-management systems are needed:
- Customer-data platform (CDP) In most companies, customer data is distributed across separate, disconnected systems typically managed by different stakeholders. A CDP solves this problem by centrally connecting the most valuable data in a flexible, unified model to develop an addressable customer identity that can be used consistently across channels. A CDP typically sits in a public or private cloud (though it can be deployed on-premise when needed) in order to handle terabytes—sometimes even petabytes—of data and make it available with low latency. A modern CDP should provide a user-friendly interface so that nontechnical marketers can use the data, plus an analytics workbench where data scientists can deploy machine learning and AI models to “signalize” the data—creating 1:1 signals and microsegments that can be used for targeting and personalization. Although the CDP must also connect with enterprise data systems throughout the organization, it should be developed as an independent, stand-alone asset, with the marketing organization defining requirements and serving as its primary user. CDP solutions range from turnkey off-the-shelf software, to fully bespoke builds, to hybrid models. We have found that a hybrid approach is often the most effective: managing an in-house data lake with an external SaaS-based CDP platform sitting on top, so to speak.
- Identity-resolution platform The CDP relies on first-party data. But in many cases, it will be impossible to resolve a customer identity using first-party data alone. Identity-resolution platforms increase the match rate of known customers with otherwise anonymous digital IDs, expanding the pool of addressable customers and prospects. Some of the techniques used include data onboarding—matching email addresses to web-browser cookies and to the unique IDs of mobile devices, as well as matching the IDs of multiple devices owned by the same individual. Identity-resolution platforms manage large-scale data partnerships that create customer, device, and location graphs across a broad ecosystem of digital and nondigital channels. Identity match rates are highly variable, depending on the vendor, types of customer segment, and geography, which is why it’s often useful to work with multiple vendors. Worth noting is that this is a space that will continue to evolve with privacy regulations. It is crucial to comply with data-privacy regulations such as GDPR in Europe, the forthcoming California Data Privacy Act, and LGPD in Brazil.
- Data-management platform (DMP) A DMP receives signalized data from the CDP and makes it available to activate in digital channels. A DMP also makes third-party data available to create additional microsegments and rich “look-alike” segments. For example, the CDP may include a sizable segment of customers who are migrating upward in value, have made more than two or more purchases in the past six months, and recently visited the website. The DMP can sync this segment against third-party data to identify subsegments within the larger group, such as millennial fashion mavens or hip, boomer grandparents. The DMP makes these microsegments available via its data marketplace, creating a much larger pool of prospects who share the same characteristics. These look-alike segments can then be targeted with tightly tailored messages and offers in owned and paid digital channels.
All these tools have been around for a while, but in most organizations, IT has traditionally taken the lead in data management. As a result, data that could be valuable for modern marketing has often been left trapped in a silo and unavailable to marketers. To break the bottleneck, marketing and IT leaders must develop a shared vision around how data can be made available across channels. It starts with the CMO and CTO/CIO working closely on a business case and road map and then rallying the needed support from across the organization. Key steps in the execution plan include detailing use cases, determining the data needed to enable them, defining how and where data will be combined, identifying the marketing technology systems that will use the data, and developing data governance and a cross-functional data-governance council.
Decisioning
Challenge: Decisioning logic resides in individual, channel-based, black-box systems—or it does not exist at all, resulting in a disjointed experience for customers.
Solution: Create an integrated decisioning engine that uses machine learning and AI models to score various propensities for each customer.
To deliver a consistent experience, savvy marketers build a set of centralized analytic models in the CDP that create propensity and uplift scores for customers at the individual level. These models allow companies to predict the next best marketing action to deliver to the customer based on a complete set of both individual and microsegment behavior. The decisioning engine needs to balance a series of competing marketing rules to ensure that offers and experiences do not collide or create inconsistent experiences. Increasingly, machine learning and complex artificial intelligence are used to optimize decisions and find patterns not previously detected by static models.
No single platform today can fully serve as a centralized decisioning engine, despite claims to the contrary. That leads some companies to build their own decisioning engine. Our experience is that this creates a lot of technical debt and potentially freezes a business out of a dynamic space. Innovative vendors are developing independent solutions, and the leading marketing cloud platforms are investing heavily to improve their AI-based decisioning capabilities.
Marketing leaders need to work with their technology colleagues to develop a solution architecture and decide how to balance what to build and what to buy. Keep in mind the trade-off between getting quick results and building long-term advantage. Key considerations include: How unique are your requirements, and can they be met with an off-the-shelf solution? How prepared and resourced is your company to build a distinctive AI-based decisioning engine and a user-friendly software interface? Are core decisioning capabilities a unique part of your value proposition and something that it is critical to own?
No matter the choice, it’s always a good idea to maintain a program of active experimentation with the new decisioning tools that are constantly emerging and improving. New vendors that offer AI-based solutions usually offer low- or no-cost trials, which provide an easy way to test new solutions versus current capabilities. This is probably the space that will evolve most in coming years.
Design
Challenge: Marketers cannot match the volume of content and pace of experimentation demanded by personalization.
Solution: Break content into small pieces that can be dynamically mixed and matched for maximum flexibility.
With personalization at scale, the number of different ways to address customers increases exponentially. This creates a need to design and create a lot more content.
To do so, a company has to move away from traditional design processes, which develop mostly bespoke content, and instead look to operating a content factory. Content—art, photos, copy, videos, emails, banners, web and app components, and even components for addressable nondigital channels—must be broken into modular components, for mixing and matching in dynamically populated templates to be delivered in multiple-form factors on the fly. All these elements need to be tagged for easy retrieval and tracking and stored as a content library inside a digital-asset-management (DAM) repository and dynamic creative-optimization platform.
The effective management of modular content and offers requires a thoughtful offer taxonomy based on a wide range of factors, including customer segment, offer type, channel, placement, product category, and related campaign. Without this taxonomy in place, offer selection cannot be automated. All content and offers must be stored and tagged using this taxonomy within a centralized offer repository, which is often a custom-developed database designed to store all offer metadata along with reference IDs that are commonly housed in the DAM. A user-friendly user interface is needed to allow nontechnical staff to create new assets and offers. Once content and offer modules have been tagged, the decisioning engine can continuously test content variations, doubling down on the versions that deliver the best results.
Distribution
Challenge: There is a lack of real-time orchestration between channels.
Solution: Integrate channels to coordinate communications and react to customer actions.
The last mile in delivering personalization at scale is connecting data, decisioning, and design elements to the marketing technology systems (such as A/B testing, content-management systems, email platforms) that deliver experiences to consumers. Having all these elements working together allows companies to react at moments of opportunity as opposed to solely relying on a series of predetermined campaigns to engage customers and prospects. For example, when a customer is shopping for a product, the data and decisioning systems identify which offer to serve based on that person’s profile, which then triggers the content and offer repository to assemble the relevant content modules and serve them to the marketing technology platforms, which in turn deliver them to the customer in the relevant channel. The system tracks how the offer performs, sending the data back to the CDP in real time so it can “learn” what works and what doesn’t
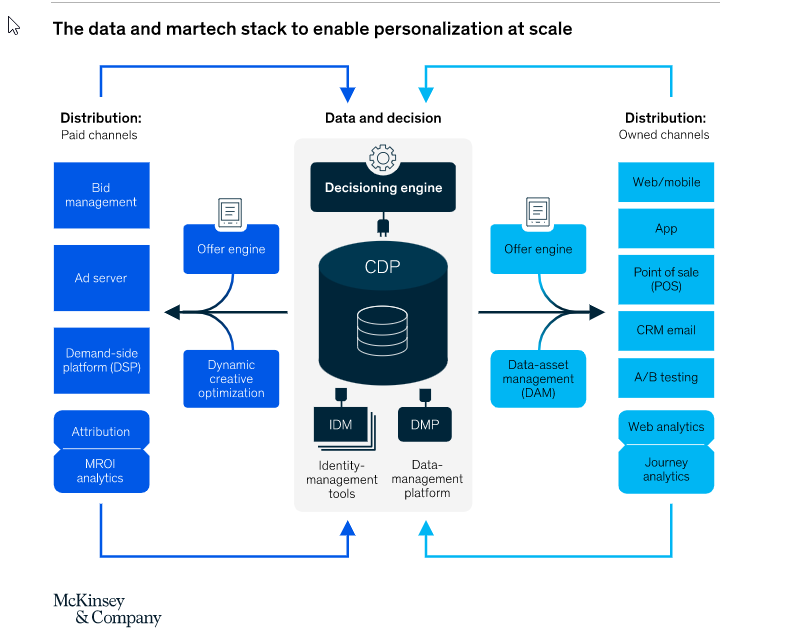
Bringing it all together
Most CDPs have application programming interfaces and other data connections that can link to the mix of channels and data systems of record (martech, adtech, CRM, content, e-commerce, and POS) that most companies use to deliver offers to customers. This makes the integration and activation of data across the tech stack relatively easy and helps to resolve a basic historical issue: marketing technology platforms that have been deployed with little thought given to how these systems could effectively share data to drive more effective engagement at every interaction across all channels.
While new technology can help resolve this problem, the bigger problem tends to be organizational. “Channel owners,” who have responsibility for a single channel, tend to be rewarded based on the performance of only that one channel. So they understandably have little incentive for taking the extra time to think about sharing data with other channels. Addressing this disconnect requires implementing an agile-marketing operating model. That means creating cross-functional teams that include channel owners and are responsible for optimizing the entire cross-channel journey of customers within their segment.
The technology to unlock the value of personalization at scale is readily available, and you may be surprised how much of it you already have in place. You don’t need to tackle everything at once. Develop a road map with regular stage gates to evaluate platform progress based on the trade-off between value at stake and ease of implementation. Identify and prioritize quick wins that make it easy for the organization to continue funding the transformation.
Lastly, remember, there are no technology issues, only people issues. People are the gatekeepers of data. People get into routines that are uncomfortable to break. People defend legacy decisions that are past their expiration date. People decide how to collaborate with their peers—and people are the spark of inspiration and agents of change who make personalization not just possible, but surprising and delightful for your customers every day
The following McKinsey article from Sean Flavin and Jason Heller provides their research perspective. here